Deer Readers
Below is an in-depth an essay that delves into the transformative concept of the AI virtual cell (AIVC), exploring its design, the integration of multi-scale and multi-modal data, successes in related fields, and the broad implications for biological research and medicine.
Abstract
Cells are the fundamental units of life, and understanding their behavior is essential to unraveling the complexities of health and disease. However, traditional experimental and computational models have struggled to capture the dynamic, interconnected nature of cellular processes. Recent advances in artificial intelligence (AI) and omics technologies have opened the door to a new era of modeling—a virtual cell powered by large neural networks. This essay explores the concept of the AI virtual cell (AIVC), examining its design principles, the integration of multi-scale and multi-modal data, examples of early successes, and its potential to accelerate discoveries in biology and medicine through interdisciplinary collaboration and open science.
1. Introduction
Cells have long been recognized as the building blocks of life, with their behavior underpinning every aspect of health and disease. Yet, traditional models, ranging from simplified biochemical networks to static representations of cellular states, have consistently fallen short of capturing the full complexity inherent in living systems. In recent years, the convergence of two revolutionary fields—artificial intelligence and omics technologies—has provided researchers with new tools capable of transcending these limitations.
At the heart of this revolution is the concept of the AI virtual cell (AIVC). An AIVC is envisioned as a comprehensive, dynamic simulation of cellular processes, built upon multi-scale and multi-modal datasets and driven by large neural networks. By representing molecules, cells, and tissues in a unified framework, AIVCs promise to transform our ability to simulate cellular function with unprecedented fidelity. This essay examines the theoretical foundations, design principles, and practical implications of the AIVC, along with real-world examples that underscore its potential to revolutionize both biological research and clinical practice.
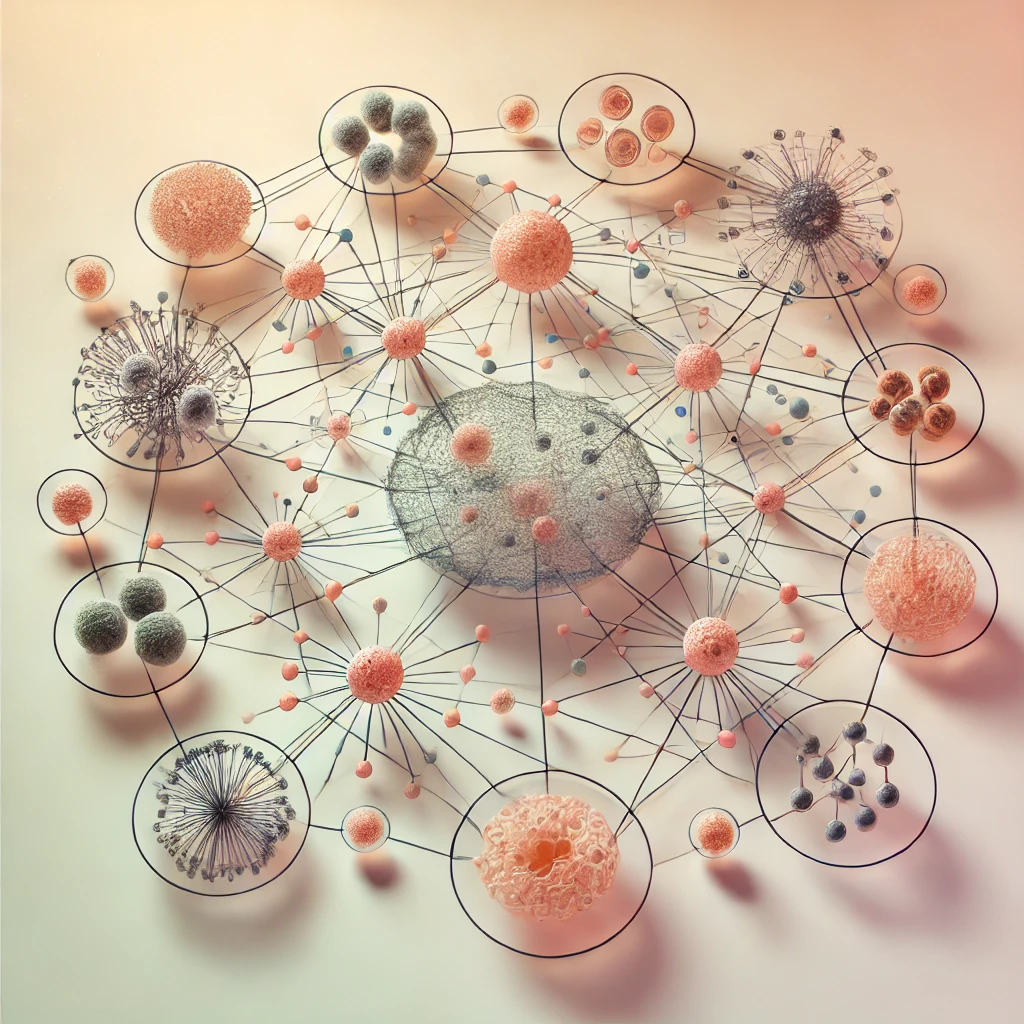
2. Limitations of Traditional Cell Models
Historically, cell biology has relied on a range of models—both experimental and computational—to study cellular functions. Traditional computational models often simplify cellular systems into discrete pathways or networks. While these models have been instrumental in advancing our understanding of cellular mechanisms, they exhibit significant limitations:
• Oversimplification of Complexity: Many traditional models reduce cellular behavior to a handful of reactions or interactions, thereby missing the broader context of cellular signaling networks and feedback loops.
• Static Representations: Conventional models typically capture cellular states as static snapshots, neglecting the dynamic, time-dependent aspects of cell behavior.
• Limited Scope: Models that focus on individual pathways fail to account for cross-talk between different cellular processes, making them inadequate for simulating responses to complex stimuli.
• Inability to Integrate Diverse Data: With the explosion of omics technologies—genomics, proteomics, metabolomics, and beyond—traditional models struggle to incorporate these diverse data types into a cohesive simulation.
These shortcomings underscore the need for more sophisticated approaches that can integrate vast amounts of heterogeneous data and simulate the dynamic behavior of cells across multiple scales.
3. Advances in AI and Omics Technologies
The last decade has witnessed remarkable advances in both AI and omics, setting the stage for a new paradigm in biological modeling.
• Artificial Intelligence and Deep Learning:
AI, particularly deep learning, has achieved breakthroughs in fields ranging from computer vision to natural language processing. In biology, deep neural networks are now being used to predict protein structures, analyze high-dimensional data, and even design new molecules. A prominent example is AlphaFold, developed by DeepMind, which achieved unprecedented accuracy in predicting protein structures from amino acid sequences. The success of such models demonstrates the capacity of deep learning to handle the complexity and variability inherent in biological systems.
• Omics Technologies:
Omics technologies have revolutionized the collection of biological data. High-throughput sequencing, mass spectrometry, and advanced imaging techniques now provide comprehensive datasets that detail the genomic, proteomic, metabolomic, and epigenetic landscapes of cells. These datasets offer a rich, multi-dimensional view of cellular states under various conditions, paving the way for their integration into dynamic, computational models.
• Integration of Multi-Modal Data:
One of the key challenges—and opportunities—in modern biology is the integration of diverse data types. Multi-modal data integration allows researchers to correlate changes at the molecular level with phenotypic outcomes, thereby providing a more holistic view of cellular function. AI models, particularly those based on large neural networks, are uniquely suited to this task, as they can learn complex patterns from heterogeneous data sources.
Together, these advances enable the creation of an AI virtual cell that leverages the full spectrum of biological data to simulate cellular behavior with remarkable accuracy.
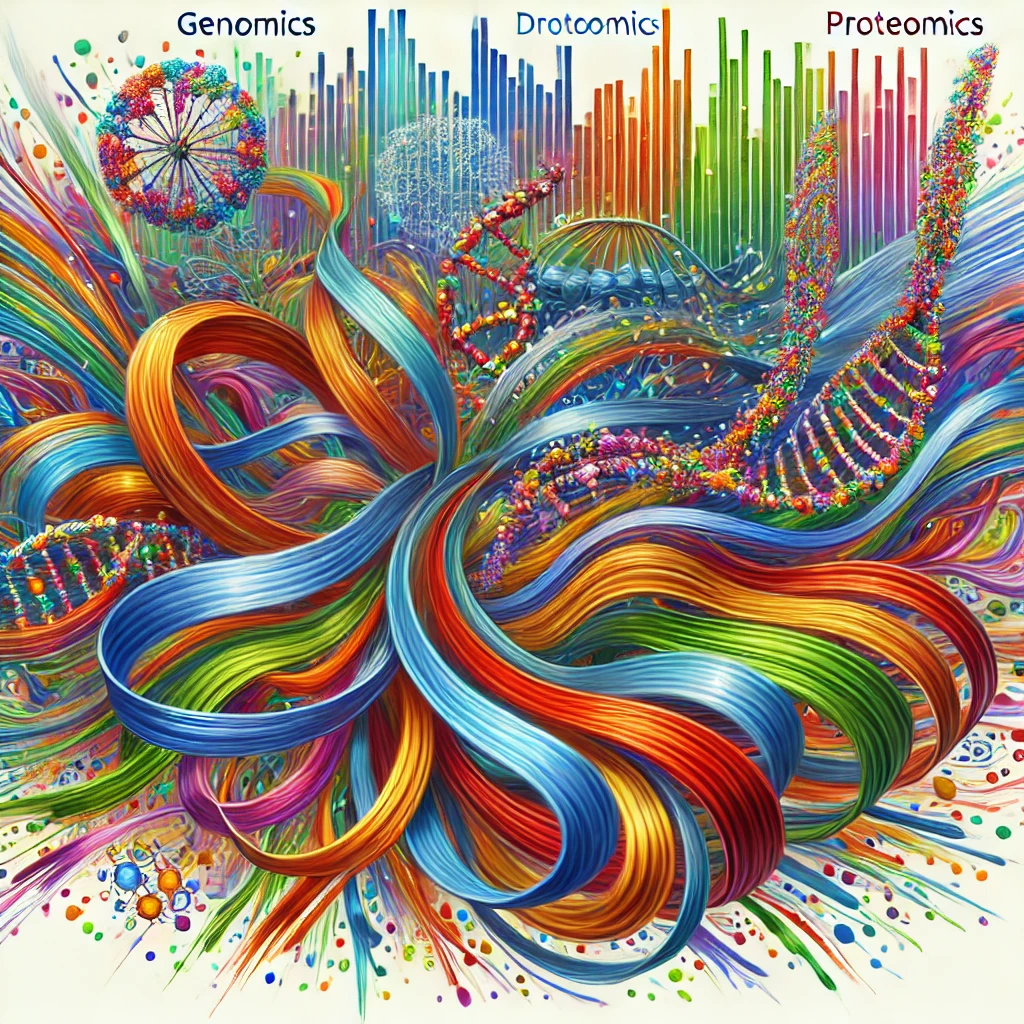
4. The Concept of the AI Virtual Cell (AIVC)
The AI virtual cell represents a paradigm shift in how we model and understand cellular processes. At its core, the AIVC is a computational framework that simulates the behavior of cells by integrating data from multiple scales—from molecular interactions to tissue-level phenomena—into a single, coherent model.
• Multi-Scale Modeling:
Cellular processes occur at various spatial and temporal scales. For example, molecular interactions such as protein binding occur on the nanometer scale and within milliseconds, while cellular responses to external stimuli can span micrometers and minutes. The AIVC is designed to bridge these scales, linking molecular-level events with larger-scale cellular and tissue behaviors. This multi-scale approach is critical for understanding how localized molecular events propagate to influence overall cell function and organismal health.
• Multi-Modal Integration:
To accurately capture the multifaceted nature of cellular processes, the AIVC incorporates diverse data types. Genomic and proteomic data provide insights into the molecular composition of the cell, while metabolomic and imaging data reveal functional states and spatial organization. By fusing these data streams, the AIVC creates a comprehensive representation of the cell that can be dynamically updated to reflect different physiological or pathological states.
• Large-Neural-Network-Based Simulation:
At the heart of the AIVC lies a deep neural network capable of learning complex patterns and interactions from vast datasets. This network functions as the engine of the simulation, predicting cellular responses to various perturbations and environmental conditions. With its ability to generalize from training data, the AIVC can simulate novel scenarios, guide experimental designs, and even predict the effects of therapeutic interventions.
In essence, the AIVC is not merely a static model but a dynamic simulation platform that can evolve as new data become available, providing a living digital twin of the cell.
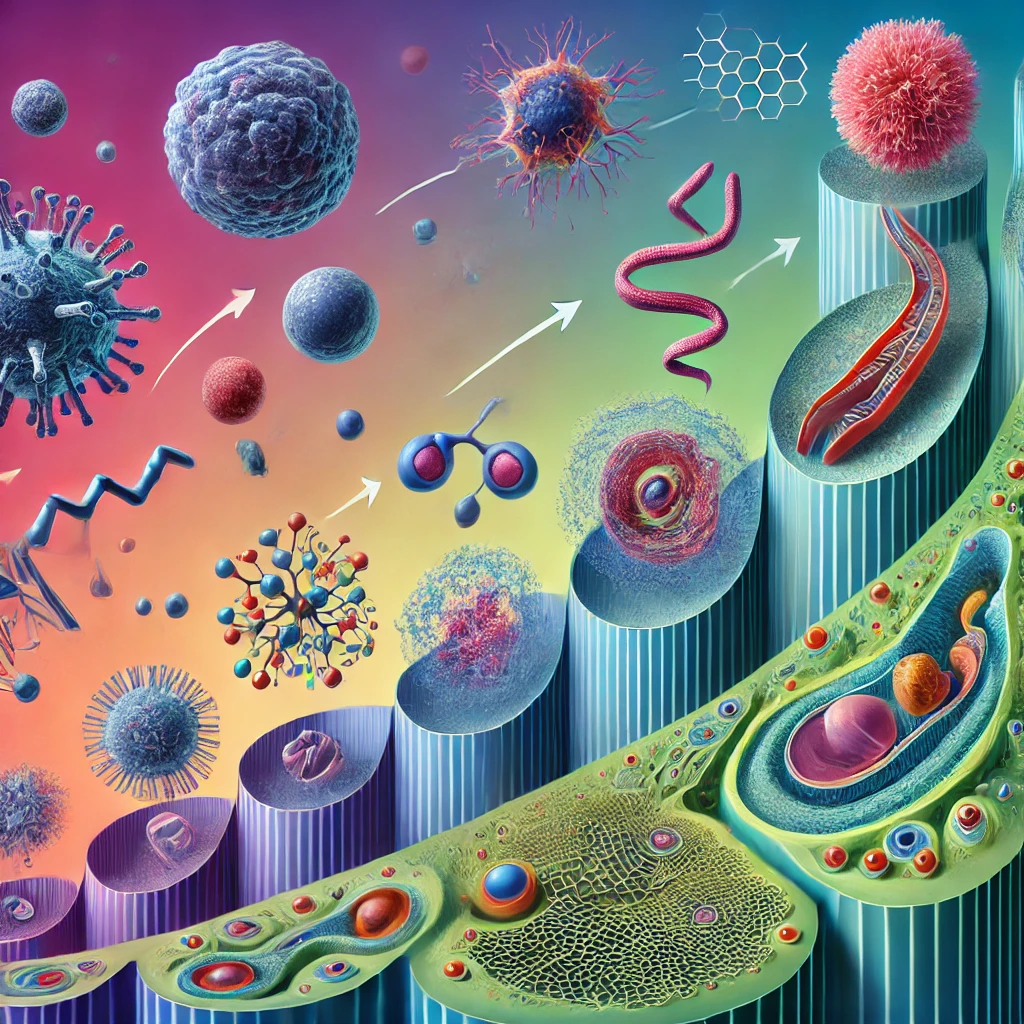
- Multi-Scale and Multi-Modal Modeling:
- Strategies and Examples A key strength of the AIVC lies in its ability to integrate data across different scales and modalities. This section explores strategies for achieving this integration and highlights several notable examples.
• Hierarchical Modeling:
One approach to multi-scale modeling is to create a hierarchical framework where molecular interactions, cellular processes, and tissue-level phenomena are represented in separate layers that communicate with one another. For instance, a molecular layer might simulate protein–protein interactions and signaling cascades, while a cellular layer models cell cycle progression and apoptosis. Such hierarchical models can be interconnected using neural networks that serve as translation layers, ensuring that events at the molecular scale appropriately influence higher-level behaviors.
• Graph Neural Networks (GNNs):
GNNs have emerged as a powerful tool for modeling relationships and interactions in complex networks. In the context of the AIVC, GNNs can represent the intricate web of molecular interactions within a cell. By treating proteins, metabolites, and other molecules as nodes in a graph, and their interactions as edges, GNNs can learn the structure and dynamics of these networks. Successful applications of GNNs in predicting protein–protein interactions and drug–target interactions underscore their potential in simulating cellular behavior.
• Case Study – AlphaFold and Protein Interaction Networks:
AlphaFold’s success in predicting protein structures has paved the way for incorporating structural data into dynamic simulations. When integrated with models that predict protein–protein interactions, researchers can simulate how structural changes influence signaling pathways and cellular responses. This approach has already been used to gain insights into the molecular mechanisms of diseases such as cancer, where misfolded or mutated proteins disrupt normal cellular signaling.
• Integration of Spatial Data:
Advances in imaging technologies allow for the capture of spatial and temporal information about cells. Techniques such as fluorescence microscopy and spatial transcriptomics provide detailed maps of where molecules are located within a cell. Incorporating these spatial data into the AIVC enables the simulation of cellular processes in their native spatial context—a critical factor in processes like cell migration, tissue regeneration, and the spread of cancer cells. Through these strategies, the AIVC aims to provide a high-fidelity simulation platform that is capable of reflecting the true complexity of living cells.
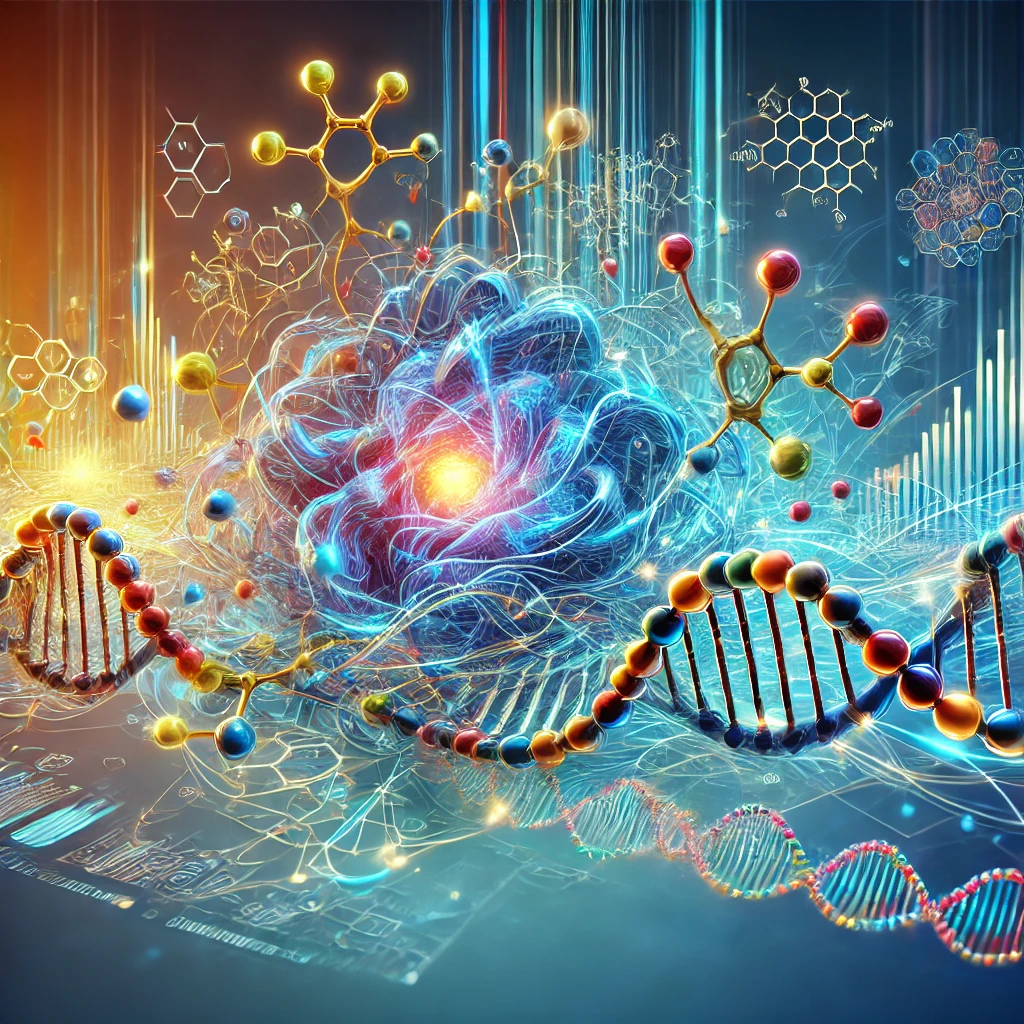
6. Successes in AI-Driven Biological Modeling
While the full realization of an AIVC is still on the horizon, numerous successes in AI-driven biological modeling provide a strong foundation for its development. These successes not only validate the underlying principles of the AIVC but also demonstrate the transformative potential of integrating AI with omics data.
• AlphaFold and Beyond:
As mentioned earlier, AlphaFold represents a monumental achievement in computational biology. By accurately predicting the three-dimensional structures of proteins, AlphaFold has solved one of the most challenging problems in structural biology. This breakthrough has enabled researchers to explore protein functions and interactions with unprecedented detail, forming a critical component of any virtual cell model.
• Simulating Metabolic Networks:
Researchers have successfully used deep learning to model complex metabolic networks within cells. For example, AI-driven approaches have been employed to predict metabolic fluxes in bacterial cells, which has important implications for bioengineering and drug discovery. These models demonstrate that it is possible to capture the dynamic interplay between enzymes, substrates, and regulatory molecules—a key aspect of cellular function.
• Predicting Drug Responses:
In oncology, AI models are increasingly being used to predict how cancer cells will respond to various therapies. By integrating genomic, proteomic, and clinical data, these models have been able to forecast treatment outcomes and identify potential drug resistance mechanisms. Such predictive capabilities are critical for developing personalized medicine approaches and highlight the potential of an AIVC in guiding experimental studies.
• Integrative Multi-Omics Analyses:
Several research groups have developed integrative platforms that combine data from different omics layers to identify biomarkers and elucidate disease mechanisms. These platforms, which often incorporate machine learning techniques, have led to the discovery of novel targets in complex diseases such as Alzheimer’s and cardiovascular disorders. The success of these integrative approaches underscores the importance of multi-modal data in creating comprehensive cellular models.
Each of these examples provides a proof of concept for the broader vision of the AIVC. They demonstrate that AI-driven models can capture complex biological processes and that integrating diverse data sources can lead to actionable insights in health and disease.

7. Implications for Health, Disease, and Experimental Research
The creation of high-fidelity AIVCs promises to have profound implications for both basic research and clinical practice.
• Accelerating Drug Discovery and Development:
One of the most immediate applications of AIVCs is in drug discovery. By simulating cellular responses to various compounds in silico, researchers can rapidly screen potential drug candidates and predict their efficacy and toxicity. This approach not only reduces the time and cost associated with laboratory experiments but also enhances the precision of therapeutic interventions. Virtual screening platforms powered by AI have already begun to influence the early stages of drug development, paving the way for more comprehensive cellular simulations.
• Personalized Medicine:
The ability to simulate an individual’s cellular behavior holds tremendous promise for personalized medicine. By incorporating patient-specific omics data into an AIVC, clinicians could predict how a patient might respond to a given therapy or how their disease might progress over time. Such predictive models could be used to tailor treatments to individual patients, thereby increasing therapeutic efficacy and reducing adverse effects.
• Guiding Experimental Studies:
High-fidelity simulations provide an invaluable tool for guiding experimental design. Researchers can use virtual experiments to test hypotheses, identify critical variables, and optimize experimental conditions before committing to costly and time-consuming laboratory work. This iterative process, where simulations inform experiments and vice versa, can significantly accelerate the pace of discovery.
• Understanding Complex Diseases:
Many diseases, including cancer, neurodegenerative disorders, and autoimmune conditions, arise from complex, multi-scale interactions within cells. Traditional models often fail to capture this complexity. An AIVC, with its ability to simulate the intricate network of interactions within a cell, offers a powerful platform for studying disease mechanisms. By revealing how perturbations at the molecular level propagate to affect cellular function, AIVCs can help identify novel therapeutic targets and strategies.
The integration of AI and omics into a unified modeling framework thus holds the potential to transform our understanding of health and disease, bridging the gap between molecular biology and clinical practice.
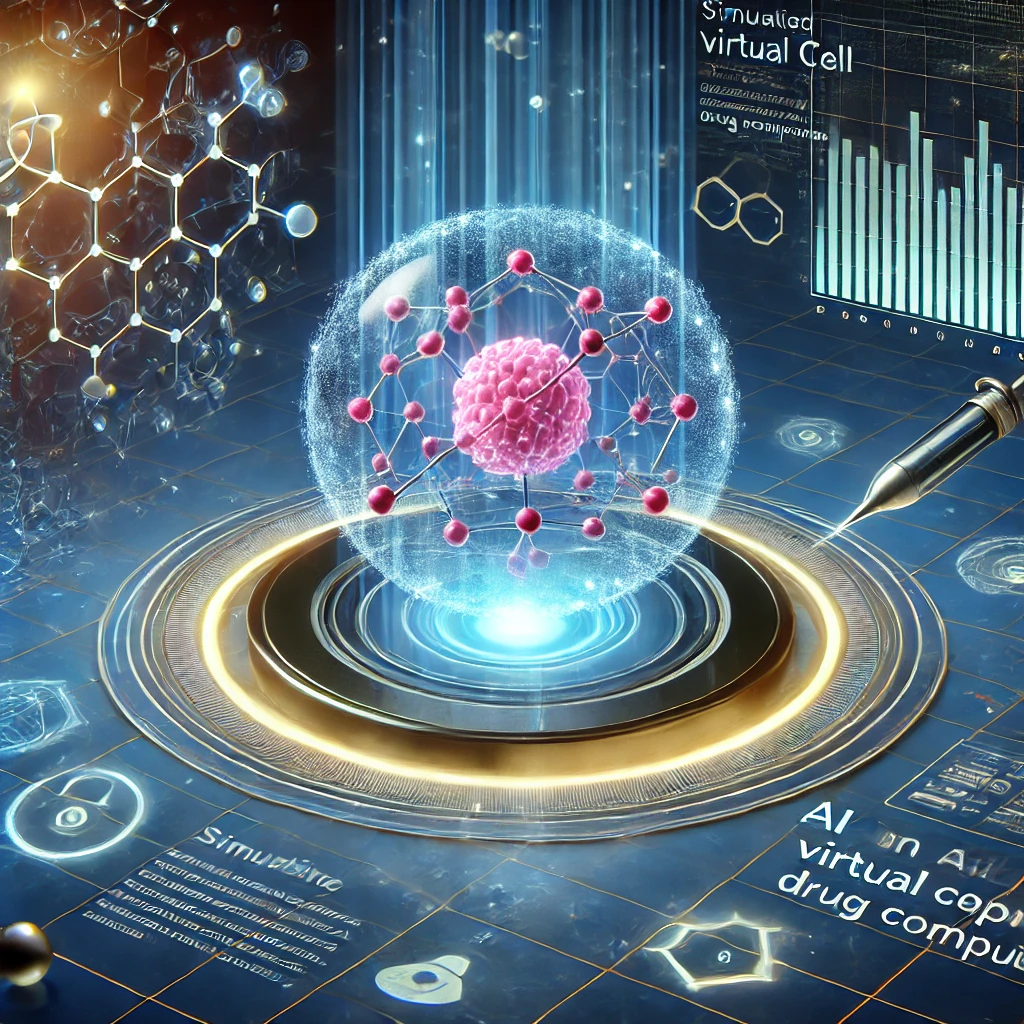
8. Fostering Interdisciplinary Collaborations and Open Science
The development and deployment of an AIVC is inherently an interdisciplinary challenge, requiring expertise from fields as diverse as molecular biology, computer science, data analytics, and engineering. Collaborative efforts are essential for several reasons:
• Combining Expertise:
Biologists bring an understanding of cellular mechanisms, while AI researchers contribute state-of-the-art algorithms and computational techniques. Engineers and data scientists, meanwhile, are crucial for managing and integrating vast amounts of omics data. Only by combining these diverse areas of expertise can a comprehensive and accurate virtual cell be realized.
• Open Science Initiatives:
Open science and data sharing are key to accelerating progress in this field. Collaborative platforms and shared databases enable researchers worldwide to contribute data, validate models, and build upon one another’s work. Such initiatives not only democratize access to cutting-edge research but also foster innovation by enabling rapid cross-disciplinary collaboration.
• Building Community Resources:
Successful projects in related fields—such as the Human Genome Project or the Cancer Genome Atlas—demonstrate the power of collaborative, open science initiatives. An AIVC project would benefit immensely from similar community-driven resources, where data, code, and methodologies are shared freely to advance collective knowledge.
By fostering an environment of collaboration and openness, the development of AIVCs can become a truly global effort, accelerating breakthroughs in our understanding of cellular behavior and disease.
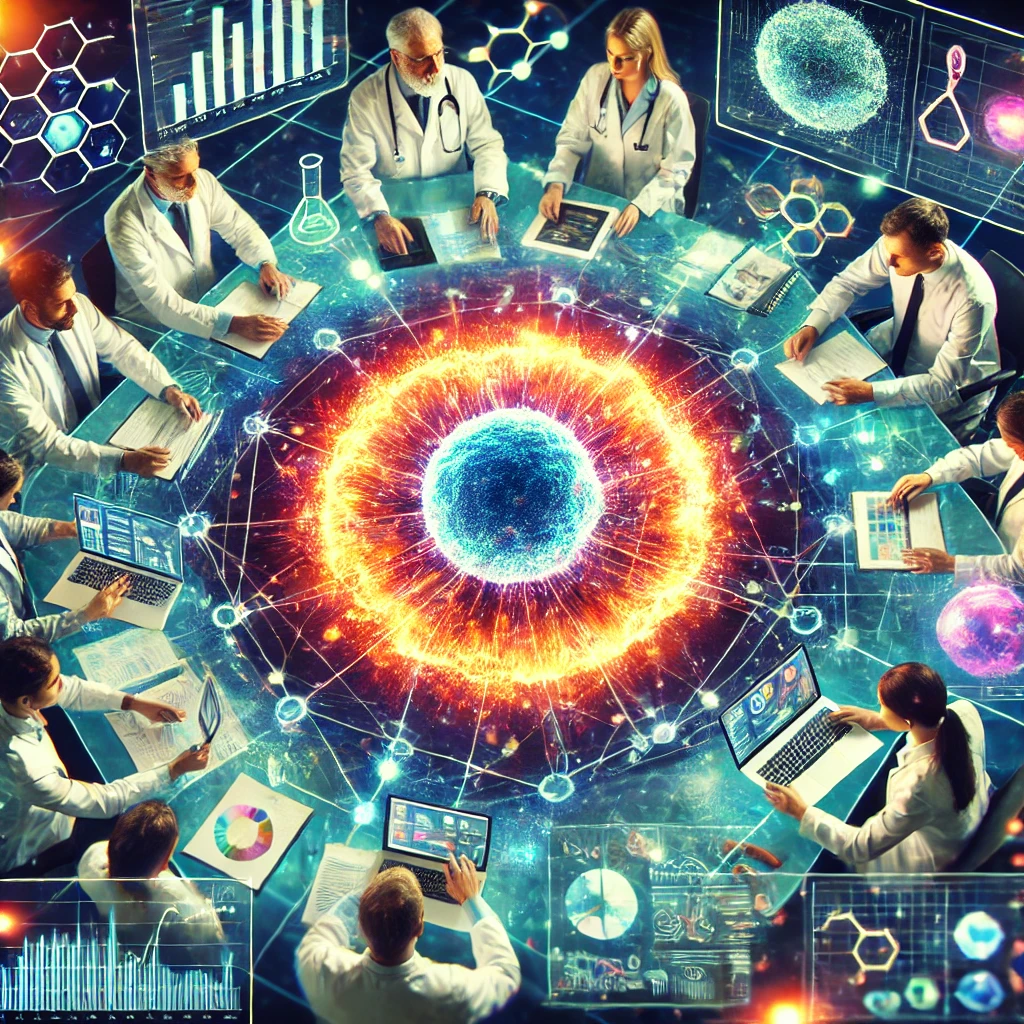
9. Future Directions and Challenges
While the vision of an AI virtual cell is compelling, several challenges must be addressed to bring this concept to fruition.
• Data Integration and Standardization:
The integration of multi-modal omics data from different sources poses significant technical challenges. Standardizing data formats and developing robust methods for data fusion will be critical for creating a coherent and accurate AIVC.
• Computational Complexity:
Simulating cellular processes across multiple scales requires immense computational power. Advances in hardware—such as high-performance computing and cloud-based infrastructures—will be necessary to support these simulations. Moreover, developing efficient algorithms that can handle the complexity of these models without sacrificing accuracy is an ongoing challenge.
• Validation and Interpretability:
As with any AI-driven model, ensuring that the AIVC produces biologically meaningful and interpretable results is paramount. Rigorous validation against experimental data is essential to build trust in these simulations. Additionally, efforts must be made to develop explainable AI techniques that allow researchers to understand how the model reaches its predictions.
• Ethical and Regulatory Considerations:
The use of patient-specific data in constructing personalized virtual cells raises important ethical and regulatory issues. Ensuring data privacy, securing informed consent, and developing regulatory frameworks for the use of AI in clinical decision-making will be necessary steps in the journey toward personalized medicine.
Despite these challenges, the rapid pace of technological and methodological advances suggests that these obstacles can be overcome. The potential rewards—a deeper understanding of cellular function, accelerated discovery, and improved patient outcomes—make this an endeavor worth pursuing.
10. Conclusion
The vision of the AI virtual cell represents a transformative approach to understanding and simulating the complex behavior of living cells. By integrating advances in artificial intelligence and omics technologies, the AIVC offers a path toward high-fidelity, multi-scale, and multi-modal simulations that capture the dynamic essence of cellular life. Early successes in related fields, such as protein structure prediction with AlphaFold and integrative multi-omics analyses, provide a solid foundation for this ambitious endeavor.
The development of an AIVC holds the promise of revolutionizing drug discovery, personalizing medical treatments, and guiding experimental research through predictive in silico models. Moreover, the interdisciplinary and collaborative nature of this project, supported by open science initiatives, ensures that the benefits of this technology will be shared across the global scientific community.
As researchers continue to overcome challenges related to data integration, computational complexity, and model validation, the AI virtual cell is poised to become a cornerstone of modern biological research. Its successful implementation will not only enhance our understanding of cellular processes but also usher in a new era of innovation in health and disease, transforming the way we study life at its most fundamental level.
In summary, the AIVC represents an exciting frontier where the convergence of AI and omics can transform our ability to model and simulate the intricate dynamics of cells. With collaborative efforts, open data sharing, and continual advancements in technology, the AI virtual cell may soon become an indispensable tool in the quest to understand the complexities of life, offering hope for breakthroughs in both research and medicine.